A recent study conducted by Dr. Chen and his collaborators at the University of British Columbia was published in the International Journal of Applied Earth Observation and Geoinformation.
Title: Remote sensing and object-based techniques for mapping fine-scale industrial disturbances.
Abstract: Remote sensing provides an important data source for the detection and monitoring of disturbances; however, using this data to recognize fine-spatial resolution industrial disturbances dispersed across extensive areas presents unique challenges (e.g., accurate delineation and identification) and deserves further investigation. In this study, we present and assess a geographic object-based image analysis (GEOBIA) approach with high-spatial resolution imagery (SPOT 5) to map industrial disturbances using the oil sands region of Alberta’s northeastern boreal forest as a case study. Key components of this study were (i) the development of additional spectral, texture, and geometrical descriptors for characterizing imageobjects (groups of alike pixels) and their contextual properties, and (ii) the introduction of decision trees with boosting to perform the object-based land cover classification. Results indicate that the approach achieved an overall accuracy of 88%, and that all descriptor groups provided relevant information for the classification. Despite challenges remaining (e.g., distinguishing between spectrally similar classes, or placing discrete boundaries), the approach was able to effectively delineate and classify fine-spatial resolution industrial disturbances.
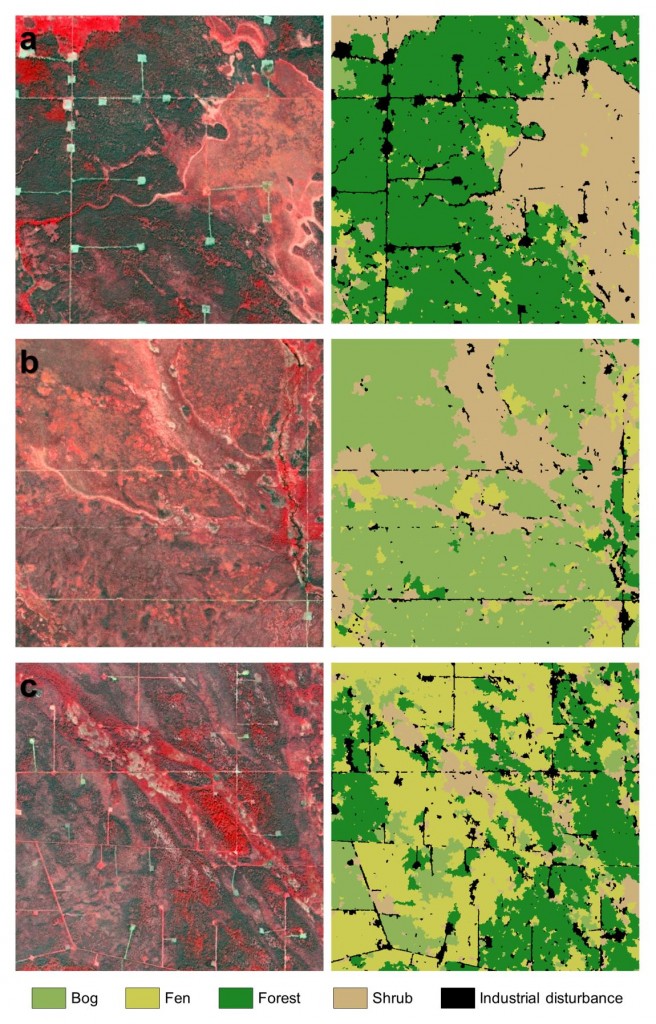
SPOT5 imagery (left) and corresponding classification (right) for locations with (a) clearly defined industrial disturbances in a forest and shrub dominated landscape;
(b) industrial disturbances in a bog and shrub dominated landscape; and (c) a fragmented landscape and many instances of industrial disturbances at various stages of
recovery. The locations a1, b1, and c1 represent areas where there was confusion observed in identifying industrial disturbances.