Abstract: Invasive plants are a major agent threatening biodiversity conservation and directly aecting our living environment. This study aims to evaluate the potential of deep learning, one of the fastest-growing trends in machine learning, to detect plant invasion in urban parks using high-resolution (0.1 m) aerial image time series. Capitalizing on a state-of-the-art, popular architecture residual neural network (ResNet), we examined key challenges applying deep learning to detect plant invasion: relatively limited training sample size (invasion often confirmed in the field) and high forest contextual variation in space (from one invaded park to another) and over time (caused by varying stages of invasion and the dierence in illumination condition). To do so, our evaluations focused on a widespread exotic plant, autumn olive (Elaeagnus umbellate), that has invaded 20 urban parks across Mecklenburg County (1410 km2) in North Carolina, USA. The results demonstrate a promising spatial and temporal generalization capacity of deep learning to detect urban invasive plants. In particular, the performance of ResNet was consistently over 96.2% using training samples from 8 (out of 20) or more parks. The model trained by samples from only four parks still achieved an accuracy of 77.4%. ResNet was further found tolerant of high contextual variation caused by autumn olive’s progressive invasion and the dierence in illumination condition over the years. Our findings shed light on prioritized mitigation actions for eectively managing urban invasive plants.
Collaborative research about urban building type mapping publishes in Remote Sensing
Urban Building Type Mapping Using Geospatial Data: A Case Study of Beijing, China
Abstract:
The information of building types is highly needed for urban planning and management, especially in high resolution building modeling in which buildings are the basic spatial unit. However, in many parts of the world, this information is still missing. In this paper, we proposed a framework to derive the information of building type using geospatial data, including point-of-interest (POI) data, building footprints, land use polygons, and roads, from Gaode and Baidu Maps. First, we used natural language processing (NLP)-based approaches (i.e., text similarity measurement and topic modeling) to automatically reclassify POI categories into which can be used to directly infer building types. Second, based on the relationship between building footprints and POIs, we identified building types using two indicators of type ratio and area ratio. The proposed framework was tested using over 440,000 building footprints in Beijing, China. Our NLP-based approaches and building type identification methods show overall accuracies of 89.0% and 78.2%, and kappa coecient of 0.71 and 0.83, respectively. The proposed framework is transferrable to other China cities for deriving the information of building types from web mapping platforms. The data products generated from this study are of great use for quantitative urban studies at the building level.
Former LRSEC student Carolynne accepts a faculty position at the University of Canterbury
Carolynne Hultquist, one of the very first students of LRSEC (2013- 2014), is currently a postdoc at Columbia University and has accepted an Assistant Professor (Lecturer) position in Geospatial Science in the School of Earth and Environment at the University of Canterbury in Christchurch, New Zealand to start June 2021.
Congratulations, Carolynne! All the best with your future endeavors.
Shadow removal paper published in RSE
Zhang, Y., Chen, G., Vukomanovic, J., Singh K.K., Liu, Y., Holden, S., & Meentemeyer, R.K. (2020). Recurrent Shadow Attention Model (RSAM) for shadow removal in high-resolution urban land-cover mapping. Remote Sensing of Environment, 247, 111945.
Abstract
Shadows are prevalent in urban environments, introducing high uncertainties to fine-scale urban land-cover mapping. In this study, we developed a Recurrent Shadow Attention Model (RSAM), capitalizing on state-of-the-art deep learning architectures, to retrieve fine-scale land-cover classes within cast and self shadows along the urban-rural gradient. The RSAM differs from the other existing shadow removal models by progressively refining the shadow detection result with two attention-based interacting modules – Shadow Detection Module (SDM) and Shadow Classification Module (SCM). To facilitate model training and validation, we also created a Shadow Semantic Annotation Database (SSAD) using the 1 m resolution (National Agriculture Imagery Program) NAIP aerial imagery. The SSAD comprises 103 image patches (500 × 500 pixels each) containing various types of shadows and six major land-cover classes – building, tree, grass/shrub, road, water, and farmland. Our results show an overall accuracy of 90.6% and Kappa of 0.82 for RSAM to extract the six land-cover classes within shadows. The model performance was stable along the urban-rural gradient, although it was slightly better in rural areas than in urban centers or suburban neighborhoods. Findings suggest that RSAM is a robust solution to eliminate the effects in high-resolution mapping both from cast and self shadows that have not received equal attention in previous studies.
Congrats Sam for successfully completing his capstone project defense
Sam Nickerson has successfully completed his capstone project and will graduate this semester. Congrats, Sam!
His research focused on analyzing the effects of small hydropower plants (SHPs) on tropical forest dynamics as compared to the large dams. He found a significantly higher rate of forest loss per megawatt produced surrounding the SHPs. He is preparing for a journal submission.
Urban forest research paper accepted by Ecological Indicators
Chen, G., Singh, K.K., Lopez, J., Zhou, Y. (in press). Tree canopy cover and carbon density are different proxy indicators for assessing the relationship between forest structure and urban socio-ecological conditions. Ecological Indicators.
Abstract
Forest canopy cover and carbon density are two pivotal biophysical parameters for assessing urban forest structure and its ecosystem services. While canopy cover (horizontal structure) has been extensively studied for understanding the relationship between socio-ecological dynamics and urban forests, carbon density (vertical structure) received little attention in the urban setting. The goal of this study was twofold: (i) exploring the differences between canopy cover and carbon density, and their relationships with socio-ecological factors across an urbanizing landscape, and (ii) assessing the effect of neighborhood category (i.e., low, medium and high development intensity) on the relationships at the neighborhood level. We used Mecklenburg County located in the Charlotte Metropolitan area of North Carolina, United States as a case study area, where rapid urban sprawl has fragmented the pine-oak-hickory dominated forests into a range of low to high housing density neighborhoods. We observed two major findings. First, canopy cover and carbon density demonstrated a generally weak correlation across various types of residential neighborhoods, although such relationship became relatively stronger in areas featuring a higher level of development intensity. Second, ecological factors (e.g., landscape spatial patterns) were found to dominate the statistical models explaining the variance in both canopy cover and carbon density compared to urban socioeconomic factors (e.g., income and age). However, the models and the explanatory factors were different for the two forest parameters, and they varied across neighborhoods of diverse development intensities. Based upon these findings, we argue that canopy cover and carbon density are different proxy indicators of forest functioning in the urban setting, and should be independently treated in urban forest management. The best management practices should be developed at the inner-city, neighborhood level, rather than the typical city level, owing to the significant, variable influence of socio-ecological conditions across neighborhood types.
Dipa successfully completed her MA defense
Dipa Dutta recently completed her MA thesis defense. Congrats, Dipa!
The title of her thesis is “Detecting plant invasion in urban parks with aerial image time series and residual neural networks (deep learning)”, where she focused on developing a fine-scale remote sensing model to detect understory invasive plant species i.e. Autumn Olive (ElaeagnusumbellataThunber) in the Mecklenburg County, NC.
Welcome Alan and Sam to join LRSEC
Alan McTaggart and Sam Holden recently started their new journey in the LRSEC (August 2019) to pursue a M.A. degree. Alan graduated from Central Michigan University with B.S. in Natural Resource Biology and Geography. Sam graduated from Appalachian State University with B.S. in Geography and Minor in Animal Studies.
Welcome aboard!
Yinan He Successfully defends his PhD dissertation
Congratulations Yinan on successfully defending his PhD dissertation!
Yinan’s novel PhD research advances the knowledge in understanding the role of interacting disturbances of wildfire and plant disease in changing forest ecosystem using multi-sensor remote sensing. He published three articles in the journal Remote Sensing of Environment, which ranks #1 in the field of remote sensing. He has accepted a postdoc position at UC Berkeley.
Yinan, best of luck with your new adventure!
New research article accepted by RSE
He, Y., G. Chen, C. Potter, and R.K. Meentemeyer (in press). Integrating multi-sensor remote sensing and species distribution modeling to map the spread of emerging forest disease and tree mortality. Remote Sensing of Environment.
Abstract:
Forest ecosystems have been increasingly affected by a variety of disturbances, including emerging infectious diseases (EIDs), causing extensive tree mortality in the Western United States.Especially over the past decade, EID outbreaks occurred more frequently and severely in forest landscapes, which have killed large numbers of trees. While tree mortality is observable from remote sensing, its symptom may be associated with both disease and non-disease disturbances (e.g., wildfire and drought). Species distribution modeling is widely used to understand species spatial preferences for certain habitat conditions, which may constrain uncertain remote sensing approaches due to limited spatial and spectral resolution. In this study, we integrated multi-sensor remote sensing and species distribution modeling to map disease-caused tree mortality in a forested area of 80,000 ha from 2005 to 2016. We selected sudden oak death (caused by pathogen P. ramorum) as a case study of a rapidly spreading emerging infectious disease, which has killed millions of oak (Quercus spp.) and tanoak (Lithocarpus densiflorus) in California over the past decades. To balance the needs for fine-scale monitoring of disease distribution patterns and satisfactory coverage at broad scales, our method applied spectral unmixing to extract sub-pixel disease presence using yearly Landsat time series. The results were improved by employing the probability of disease infection generated from a species distribution model. We calibrated and validated the method with image samples from high-spatial resolution NAIP (National Agriculture Imagery Program), and hyperspectral AVIRIS (Airborne Visible/Infrared Imaging Spectrometer) sensors, Google Earth® imagery, and field observations. The findings reveal an annual sudden oak death infection rate of 7% from 2005 to 2016, with overall mapping accuracies ranging from 76% to 83%. The integration of multi-sensor remote sensing and species distribution modeling considerably reduced the overestimation of disease effects as compared to the use of remote sensing alone, leading to an average of 26% decrease in detecting disease-affected trees. Such integration strategy proved the effectiveness of mapping long-term, disease-caused tree mortality in forest landscapes that have experienced multiple disturbances.
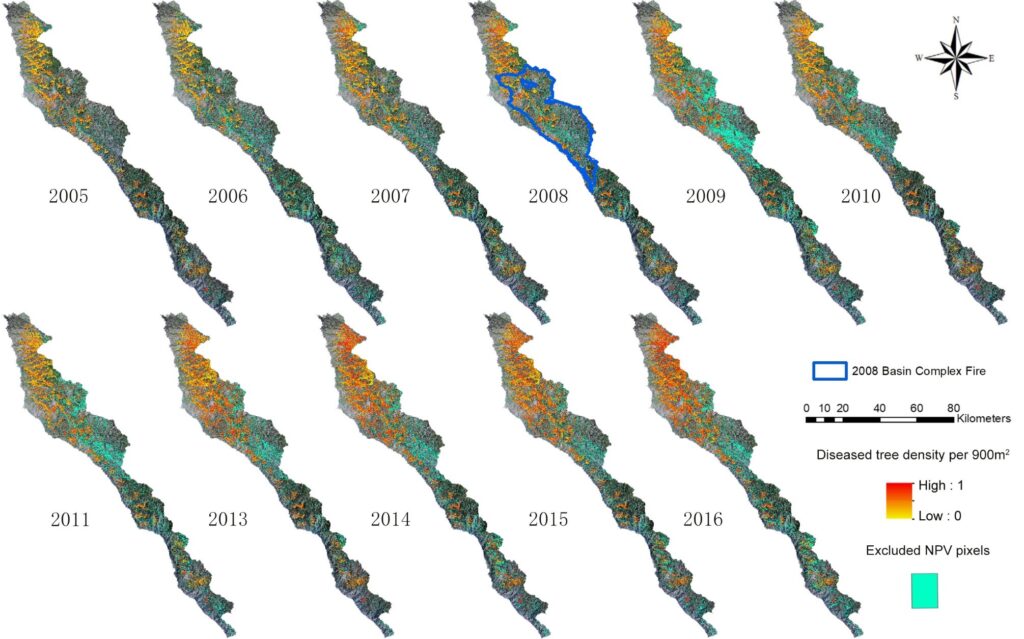