A team led by Dr. Chen and researchers from NC State, UC Davis and Lewis & Clark College recently published their new findings in ISPRS Journal of Photogrammetry and Remote Sensing.
Title: Object-based assessment of burn severity in diseased forests using high-spatial and high-spectral resolution MASTER airborne imagery
Abstract: Forest ecosystems are subject to a variety of disturbances with increasing intensities and frequencies, which may permanently change the trajectories of forest recovery and disrupt the ecosystem services provided by trees. Fire and invasive species, especially exotic disease-causing pathogens and insects, are examples of disturbances that together could pose major threats to forest health. This study examines the impacts of fire and exotic disease (sudden oak death) on forests, with an emphasis on the assessment of post-fire burn severity in a forest, where trees have experienced three stages of disease progression pre-fire: early-stage (trees retaining dried foliage and fine twigs), middle-stage (trees losing fine crown fuels), and late-stage (trees falling down). The research was conducted by applying geographic object-based image analysis (GEOBIA) to MASTER airborne images that were acquired immediately following the fire for rapid assessment and contained both high-spatial (4 m) and high-spectral (50 bands) resolutions. Although GEOBIA has gradually become a standard tool for analyzing high-spatial resolution imagery, high-spectral resolution data (dozens to hundreds of bands) can dramatically reduce computation efficiency in the process of segmentation and object-based variable extraction, leading to complicated variable selection for succeeding modeling. Hence, we also assessed two widely used band reduction algorithms, PCA (principal component analysis) and MNF (minimum noise fraction), for the delineation of image objects and the subsequent performance of burn severity models using either PCA or MNF derived variables. To increase computation efficiency, only the top 5 PCA and MNF and top 10 PCA and MNF components were evaluated, which accounted for 10% and 20% of the total number of the original 50 spectral bands, respectively. Results show that if no band reduction was applied the models developed for the three stages of disease progression had relatively similar performance, where both spectral responses and texture contributed to burn assessments. However, the application of PCA and MNF introduced much greater variation among models across the three stages. For the early-stage disease progression, neither band reduction algorithms improved or retained the accuracy of burn severity modeling (except for the use of 10 MNF components). Compared to the no-band-reduction scenario, band reduction led to a greater level of overestimation of low-degree burns and underestimation of medium-degree burns, suggesting that the spectral variation removed by PCA and MNF was vital for distinguishing between the spectral reflectance from disease-induced dried crowns (still retaining high structural complexity) and fire ash. For the middle-stage, both algorithms improved the model R2 values by 2% to 37%, while the late-stage models had comparable or better performance to those using the original 50 spectral bands. This could be explained by the loss of tree crowns enabling better signal penetration, thus leading to reduced spectral variation from canopies. Hence, spectral bands containing a high degree of random noise were correctly removed by the band reduction algorithms. Compared to the middle-stage, the late-stage forest stands were covered by large piles of fallen trees and branches, resulting in higher variability of MASTER imagery. The ability of band reduction to improve the model performance for these late-stage forest stands was reduced, because the valuable spectral variation representing the actual late-stage forest status was partially removed by both algorithms as noise. Our results indicate that PCA and MNF are promising for balancing computation efficiency and the performance of burn severity models in forest stands subject to the middle and late stages of sudden oak death disease progression. Compared to PCA, MNF dramatically reduced image spectral variation, generating larger image objects with less complexity of object shapes. Whereas, PCA-based models delivered superior performance in most evaluated cases suggesting that some key spectral variability contributing to the accuracy of burn severity models in diseased forests may have been removed together with true spectral noise through MNF transformations.
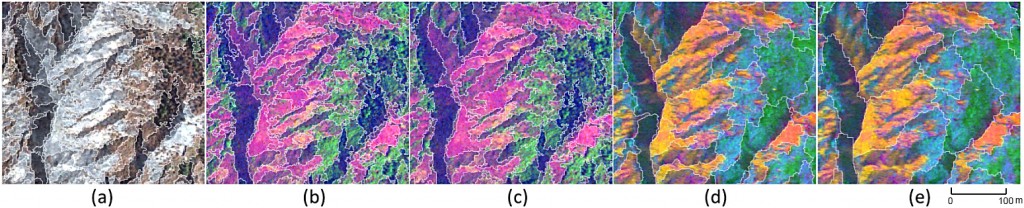
Sample objects (with white polygon boundaries) derived from five band-reduction scenarios: (a) no band reduction (i.e., 50 bands), (b) 5 PCA components, (c) 10 PCA components, (d) 5 MNF components, and (e) 10 MNF components, overlaid on images with RGB composites of (a) bands 5, 3, and 1, and (b-e) components 1, 2, and 3. The backdrop of (a) is a true color composite using MASTER bands 5, 3, and 1, where light tones represent ash, brown colors indicate moderate burns in forest stands, and a small portion of green trees reveal low level of fire damage. The backdrops of (b) and (c) are the color composites using the first three PCA components, while the backdrops of (d) and (e) are the color composites using the first three MNF components.